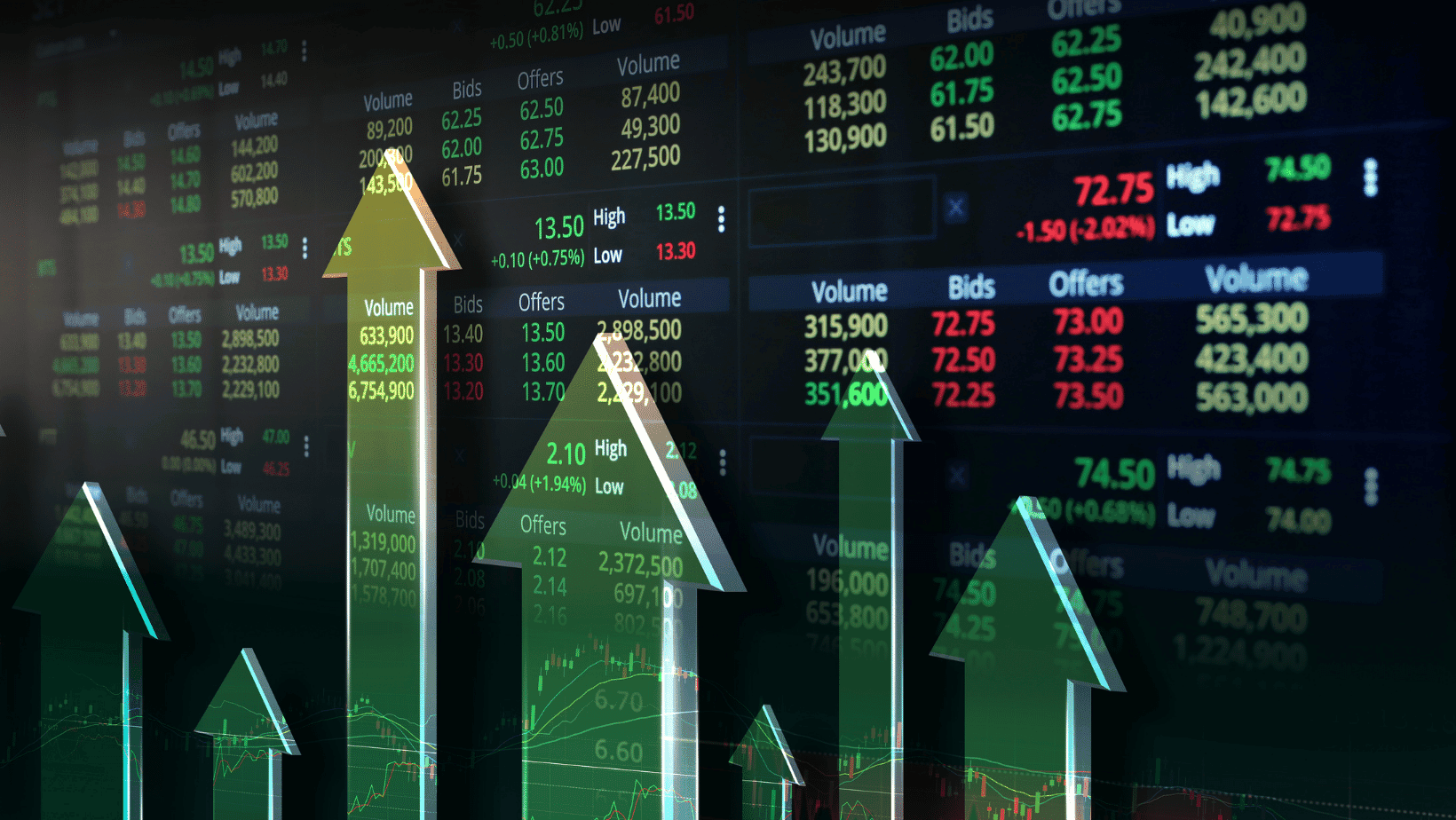
In the realm of stock trading, Dollar-Cost Averaging (DCA) stands out as a popular investment and trading strategy, offering a disciplined approach to buying assets over time.
Among its variants, two prominent methodologies emerge: time-based and data-driven models. While both aim to capitalize on market fluctuations, the data-driven model holds a distinct advantage over its time-based counterpart, owing to its adaptive nature and focus on technical indicators.
The time-based DCA strategy operates on a fixed interval, typically buying stocks on predetermined dates, such as monthly or quarterly. Conversely, the data-driven model leverages technical indicators and market signals to confirm purchase decisions. By analyzing factors like price trends, volume movements, and momentum indicators, this approach strategically times stock purchases to coincide with favorable market conditions.
Often times, data-driven model is implemented using a stock trading bot.
One of the key strengths of the data-driven DCA model lies in its ability to optimize entry points based on market dynamics. Unlike the time-based strategy, which adheres strictly to a predefined schedule, the data-driven approach adapts to fluctuations in stock prices and indicators. By purchasing stocks when technical indicators signal opportune moments, investors stand to benefit from better averaging down of the price point, potentially enhancing overall returns.
A notable advantage of the data-driven model is its propensity to capitalize on corrective price movements. When technical indicators align with buying conditions during market corrections, investors employing this strategy can acquire shares at lower prices, effectively reducing the average cost per share over time. In contrast, the time-based approach may lead to purchases regardless of whether the stock is at a high or low point, overlooking opportunities for more advantageous entry points.
Moreover, the data-driven DCA strategy fosters a dynamic trading approach that responds to market fluctuations in real-time. By incorporating technical analysis into decision-making processes, investors can adapt their buying patterns to align with evolving market conditions and emerging trends. This flexibility allows for greater precision in timing purchases, potentially enhancing portfolio performance and mitigating risks associated with market volatility.
While both time-based and data-driven DCA strategies offer systematic approaches to stock investing, the latter holds distinct advantages in its adaptability and focus on market indicators.
By harnessing the power of technical analysis, investors can optimize entry points, capitalize on corrective price movements, and navigate changing market dynamics with greater precision.
In the pursuit of maximizing returns and minimizing risk, the data-driven DCA model stands as a compelling choice for savvy investors seeking to unlock the full potential of their investment portfolios.
Traders and investors can consider a stock trading bot software like StockHero to help implement the data-driven DCA model.
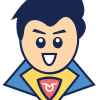